News
What’s next for CID?
With this question in mind researchers joined our meeting on 3 November. In the plenary portion we set the tone with CID project updates as well as a progress update for the CD2 metadata project by Otto Lange. Both highlighted progress and plans, not just scientifically but also in terms of sharing information, expertise and results. Our purpose was to hear what CID researchers themselves had to say about CID’s future. So most time was spent in small group discussions about future themes.
In September we asked CID researchers what future themes they discerned. We were overwhelmed with great suggestions. In fact, when we asked your help whittling down the themes by picking a top two, most send in more than two favourites. We very much appreciated the help of CID researchers in this process which resulted in five different themes that were discussed by 34 researchers across seven tables. Based on their notes, here are summaries (including some great takeaways) per theme:
Causality modelling
Nine researchers spread across two tables.
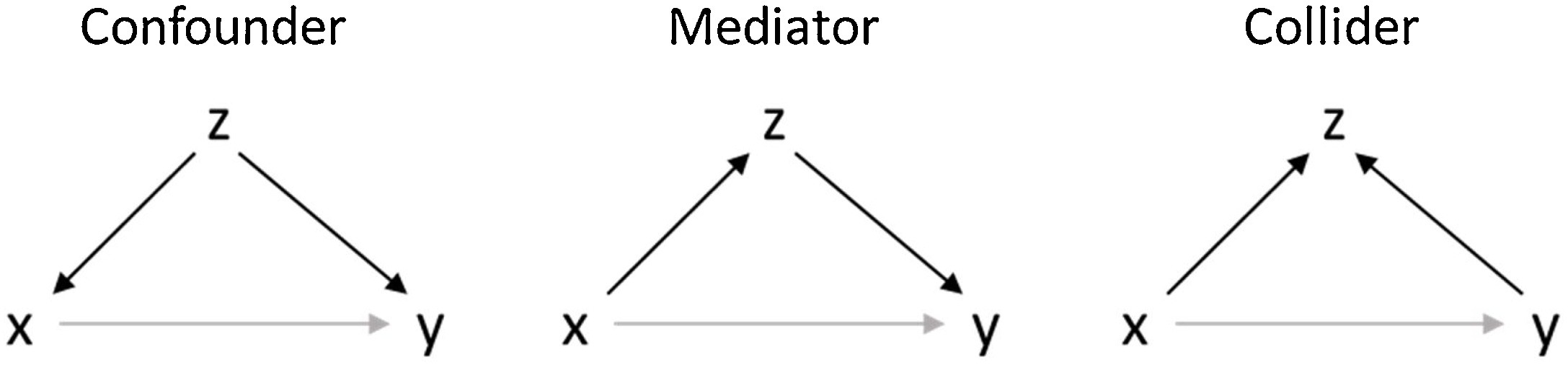
Current status
Many CID researchers appear to be interested in causality and it plays a big role in research questions. It turned out that causality may not be as intuitive as researchers think. There are many different ways to look at causality. Scientific fields also have different solutions and for CID it is relevant to include an interdisciplinary perspective. There are several needs within CID:
- appropriate plans of analyses that will increase the possibility of “more causal” relationships being drawn
- more clarity on how different perspectives relate to each other
- interdisciplinary collaborations / try to translate methods from other disciplines to e.g. social sciences
Next steps
This led to suggestions:
- More consultation opportunities for applied researchers from statisticians before they start their project and during their project
- We need to draw connections between disciplines and understand how other fields are addressing causality. Then translate this to our own field in an understandable way.
- Thinking more critically beforehand about measures in your study and the factors you are interested in
Bearing in mind potential caveats, such as:
- Even the most sophisticated models make assumptions
- In longitudinal studies the phenotype can change a lot (for example the words that infants produce). How do you ensure that you assess the same behaviour (with a changing phenotype) over multiple timepoints?
- X can predict Y at one time point, but this relationship may be different at another time point (example: heart rate goes up from exercise, but can also go down from exercise in the long run)
Key takeaways
It is complicated! Causality is an important question within CID and it is important to think about how we can actually infer causality from the data, if we can at all. As causality is at the core of the aims of CID, more discussion/ workshops on the topic could be useful. Also, CID might benefit from better “advertising” the availability of the statistics people within CID, so that CID researchers realize that statistics consultation around causality is available. To that last point we would like to add that work package 4 includes several statisticians. People such as Jeroen Mulder (whose CID PhD project revolves around causal inferences) are just an email away.
Harmonizing information and resources across cohorts
Five researchers at one table.
Current status
As part of a wider general discussion in science, it is clear harmonizing information and resources is important. Researchers highlighted the relevance of harmonization to foster collaboration, increase the power to answer relevant research questions and potentially tackle complex questions about causality. This theme is also very current and relevant to CID because we recently received funding to build a (digital) metadata infrastructure. This is a great opportunity to compare (meta)data and concepts across cohorts.
Next steps
For the metadata project Otto Lange already highlighted next steps to achieve a harmonized digital infrastructure of metadata in his presentation. This will involve a variety of experts in the fields of IT, science and data to achieve convergence on data, software, vocabularies, and potentially even different theories and concepts held by researchers about their subject matter.
There are other steps that can be taken as well. Although ideally constructs are measured in exactly the same way across all cohorts, heterogeneity can be expected in already collected data. For existing data there are statistical ways to deal with heterogenous data. It would also be good to create so called “reference datasets” by comparing all the tools used by the cohorts in a subgroup of participants. Of course in a next wave of data collection, it would we worthwhile to try to collect data, for instance biomaterial, uniformly. To do this we need to document the protocol and make it widely available.
Key takeaways
For the future, harmonizing data acquisition and analyses across CID-cohorts for essential measures will improve power and replication. The cohorts should also increase collaboration, particularly prospectively rather than retrospectively (as by necessity was the case when we started out). Collaboration can move beyond outcomes and instruments, to also include study design and infrastructure (e.g. collaboration for biomarker collection and storage).
Intergenerational transmission
Six researchers at one table
Current status
CID work package 3 focuses on intergenerational transmission. As part of CID, three WP3 cohorts (RADAR, NTR and TRAILS) are currently including children of the participants they were already tracking (third generation). Now that the different cohorts have sufficient data to provide new insights, it is interesting to think about where to go next. From the current ongoing work several things stand out:
- It is difficult to pinpoint causality and genetics. It is often overlooked as confounder (also see recent paper “Nurture might be nature: Cautionary tales and proposed solutions” by Elsje van Bergen)
- In other countries it is more common to study subgroups who are at risk
- Break cycle of transmission: study which children benefit from interventions
Next steps
Supplement the three-generations intergenerational transmission studies with small intervention or experimental studies (to obtain detailed “short-movies” in between the large “snapshots”). That will be helpful in learning about mechanisms or processes of transmission, including:
- their robustness over time,
- which developmental periods are salient for transmissions,
- which experiences mediate or moderate such transmissions,
- which genetics play a role during different stages of development and transmission
Currently perceived obstacles include privacy regulations (AVG), cohort effects that need controlling, integrating different data types/sources and potential stigmatization of hard-to-reach groups.
Key takeaways
There is a lot of potential to examine intergenerational transmission, for instance by combining various datasets and including genetic material to address issues concerning causality. But there is still a long way to go. We need more collaboration with three-generation cohorts within and outside CID, to attract more lower SES participants, and develop ethical and analytical procedures to use existing data better.
New approaches to model development utilizing the enormous amount of data already collected
Nine researchers spread across two tables.
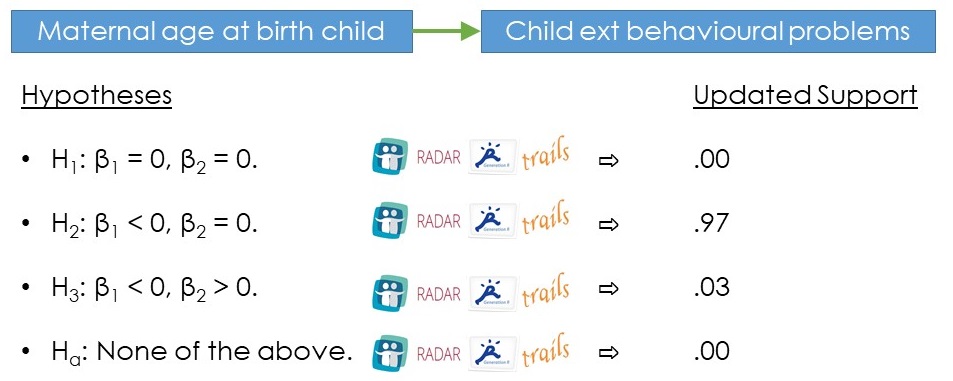
Bayesian statistics is one innovative approach already applied by CID researchers to combine cohorts (more information about this method as provided by statistician Mariëlle Zondervan-Zwijnenburg here)
Current status
Both groups emphasized the importance of the metadata catalogue currently being developed. This overview of the available data in all CID cohorts will be relevant for a broad range of researchers, also outside of CID.
There are also existing methods to combine data from the different cohorts:
- Bayesian evidence synthesis (even if data is a not exactly the same) (more information here)
- Meta-analyses (requires more similarity in data)
- Machine learning (for prediction and improvement of prediction)
Next steps
Once it is clear how much data is available (through the metadata catalogue), we can (1) identify external data sources with additional information (e.g. record linkage) and (2) start using existing methods. It is important to get feedback from researchers on the catalogue, but also to link animal databases to human databases for more collaboration and interaction. For this approach to be successful maintenance and availability of the metadata catalogue after CID ends is important.
Another step to take is to organize expert meetings/speed date sessions to talk with a small group with similar interests/problems about what method will be best to approach a specific research question. Also to raise awareness of potential pitfalls to new methods such as machine learning. For instance the possibility of double dipping. Namely, allowing the data to be both used for exploratory as for confirmatory analysis. This could possibly bias later outcomes.
Key takeaways
The CID consortium can offer unique opportunities both for exploring data across cohorts, as well as for confirming standing theories and studying their reproducibility. In the upcoming years it is important to collaborate between cohorts and datasets to answer the larger developmental questions. The metadata catalogue is an important step to obtain insight in the available data, but we also need to share expertise and stimulate interdisciplinary cross-talk and collaborations. New methodologies often have advanced technical aspects so we need to tread lightly.
Youth culture: (social) digital media use among children
Five researchers at one table.
Current status
Social media use has risen rapidly and receives (new) wide spread attention through documentaries like “the Social Dilemma”. But the effect of (social) digital media on people can be hard to define, and ranges from very negative (decreased attention spans) to more positive (increased social networks). This triggered the question:
“What is the role of digital media in the development of young children and adolescents and to what degree are we able to already investigate this in CID and can we add concepts to cohorts in the future?”
Next steps
The next step would be to form a focus group on (social) digital media and development. We would like representatives of every cohort to discuss how we can combine data already gathered on (social) digital media use within the different cohorts, and what we can do more. Representatives could be anyone interested in the topic, or with knowledge on what is being gathered on information within the cohort she/he is affiliated with.
Several potential (research) ideas:
- A (virtual) discussion with teenagers to assess their social media use and the up- and downsides.
- Teenagers may be aware of the upsides (social network) and downsides (you need meaningful, “real-life” friendships) of social media use, but are they aware of the effects that algorithms can have on the content they see online and their thoughts and views?
- (social) digital media use and brain development
- (social) digital media use and attention development
- Might be able to couple outcomes of social competence and behavioural control to daily challenges of adolescents such as social media use. The metadata initiative is a good place to start as it will catalogue overlapping questionnaires across cohorts.
Key takeaways
Social media use is a timely important topic when it comes to child and adolescent development. Therefore there is a call to start a focus group with representatives from different cohorts to inventorize and brainstorm on what we can do with data already gathered on (social) digital media use, and what we can do more of. If you want to join, please contact Yentl de Kloe.